By
Matt Dixon
|
Date Published: August 07, 2019 - Last Updated June 28, 2022
|
Comments
An IndustryVoices post.
One of the questions I’ve found myself asking customer service and customer
experience leaders in recent years is whether they’re happy with the
results they’re seeing from their post-call survey.
To be clear, I’m not asking about whether their key metrics are pointed in
the right direction, I’m asking whether they’re happy with the survey itself as a means of collecting customer feedback, measuring the
performance of their team and spotting opportunities for improvement.
Almost universally, the answer I most frequently get back is along the
lines of “Not really, but I’m not sure what other options we have.”
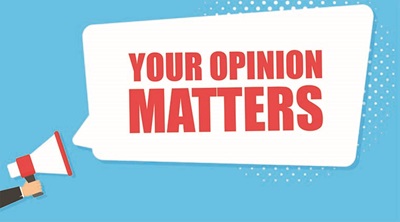
Companies have a love-hate relationship with their post-call surveys—and
it’s a relationship these days that is skewing much more negative than
positive. As almost any CX or service leader will tell you, survey response
rates are on a secular decline—in large part because companies over-rely on
surveys to answer all manner of questions, resulting in customers
experiencing survey fatigue and, ultimately, tuning them out. We hear from
companies regularly that their response rates are plummeting—in some cases,
dropping by half in just the past year.
In response to falling response rates, companies have taken the step of
shortening their surveys—today, it’s not uncommon to see surveys with only
one numerical question with an open-field text box asking for more color
(e.g., “Why did you give us the score that you did?” or “What can we do to
improve?”). While shorter surveys may temporarily stop the bleeding on
response rates, they have the unintended effect of also diminishing the
quality of the feedback that’s received—and this is to say nothing of the
well-documented biases (e.g., recall bias and extreme response bias) that
plague surveys as a VoC instrument.
These shortcomings are much more than just a nuisance to deal with—they
create real problems for leaders. Low response rates make it hard to
credibly use survey scores as a way to measure performance (especially
rep-level performance). Low response rates and systemic response bias also
raise hard questions about whether improvement opportunities surfaced
through surveys are really that widespread and worth the time and
investment to pursue. And when the verbatim collected in surveys is
lacking, leaders don’t get the why behind the what—in
other words, they may know the experience is falling short in the eyes of
customers, but they don’t know what to do to fix it.
The irony, of course, is that the technology exists for companies today to not have to rely on surveys to answer many of the most important
questions they have about the customer experience.
Recent advances in areas like automated speech recognition, natural
language processing and machine learning have made it possible for
companies to leverage data they already have—namely, recorded
phone calls, chat interactions, etc.—to predict survey scores, obtain rich
customer feedback and finally lower their reliance on surveys.
What if, instead of deploying surveys to customers after the fact, you
could harness these technologies to understand all of your recorded
conversations and predict the score a customer would have given on
a survey without having to ask the customer to fill out the survey at all?
If you could do this, you’d have no more response rate challenges since you
could assign a score to every call, not just the ten percent of
customers (or fewer) who fill out the survey. You’d have no bias issues
because you’d be working off of the raw conversational data (not a post-hoc
interpretation of what happened). And, best of all, you’d have an
incredibly rich, actionable data set to work with (i.e., no more trying to
decipher what the customer meant by “You guys rock!” or “You guys are the
worst!”).
A few years ago, this sort of thing might have felt like science fiction,
but as I learned recently, it’s very real.
Our data science team at Tethr recently took this challenge on and we were
pretty amazed by the results. To build our predictive model, our data
science team first had to decide what the outcome metric was that we wanted
to study. Since so much of what we do for care and CX leaders is help them
identify and eliminate sources of customer effort (and because I was a
co-author of from
The Effortless Experience), it seemed like a natural thing to do would be to see if we could
predict Customer Effort Score.
Before we get into how we built our model, it might make sense to provide a
bit of background on the Customer Effort Score for readers who may not be
familiar with it.
In 2008, the research team I was leading at CEB (now, Gartner) discovered a
new customer experience metric that we called the Customer Effort Score, or
CES for short. We found that this measure proved to be more highly
correlated with loyalty attitudes and behaviors like repurchase, share of
wallet and advocacy than metrics like Net Promoter Score (NPS) or Customer
Satisfaction (CSAT)—especially when applied in a transactional environment
like customer service.
The original CES was a survey question that asked customers how much effort
they had to put forth to get their issue resolved. Customers rated their
experience on a 1-5 scale where 1 was low effort (i.e., good) and 5 was
high effort (i.e., bad). By collecting CES scores from customers, companies
would be able to zero in on those interactions and experiences that
customers deemed to be “high effort,” thereby helping to surface
improvement opportunities like training or coaching, QA scorecard changes,
process fixes and website overhauls.
When we released the book, The Effortless Experience, in 2013, we
unveiled a new version of the score which we called “CES 2.0.” We found
that some companies felt the original question (“How much effort did you
personally have to put forth to handle your request?”) could cause some
customer confusion…and the term “effort” was hard to translate into certain
languages. The new question asks the customer to respond on a 1-7 scale,
from “strongly disagree” to “strongly agree,” with the statement “The
company made it easy for me to handle my issue.” Not only is the wording
more straightforward for customers and easier to translate from the
original English, but the 1-7 scale and the fact that a low score was now
“bad” and a high score “good” is more in keeping with the way other survey
questions (like CSAT or Net Promoter) are asked.
While this new version of the CES question improved on the original, it
didn’t solve for what is a more fundamental problem:
relying on surveys to ask a question that companies should already know
the answer to.
To create our predictive Customer Effort model, our data science team took
tens of thousands of completed post-call surveys (surveys in which the CES
question was asked) from a wide range of companies and industries and then
mapped those surveys to the actual calls that preceded them. In simple
research terms, the CES score the customer gave was our “dependent”
variable (i.e., the thing we were trying to predict) and everything that
happened in the preceding call served as a set of “independent” variables
(i.e., the things that might affect the dependent variable).
One of the cool things about using recorded conversational data is that
it’s a far richer data set than what we had access to in the
original Effort research, which was all based on survey data. In a survey,
there’s a natural limit to how much you can ask before a respondent gets
impatient and bails out. For instance, in the original Effort research, we
asked about channel switching (i.e., did a customer first go to the
company’s website, give up and then pick up the phone to call?). As much as
we would have liked to ask dozens of questions about the actual website
experience (e.g., was it a login issue, an unclear FAQ, confusing
information in an expert community or something else that caused the
customer to give up?), we also wanted people to fill out the survey so that
we could do the analysis.
With conversational data, however, this isn’t an issue. On the phone,
customers will go into incredible depth about exactly what went wrong in their experience. Customers won’t just
tell you it was a Website issue, but will tell you it was a login issue and
what the specific error message was that they received. They won’t just
tell you they found the content on the Website confusing. They’ll tell you
which specific FAQ was confusing to them and why. With all of this rich,
contextual data, our team was able to generate a truly massive list of
potential variables that we could measure.
So, using conversational data allowed us to cast a really broad
net, in other words.
And casting a broad net is important because Effort, we’ve learned, isn’t
something that can easily be reduced to a survey score. It’s nuanced—a
condition that is comprised of many things with many flavors. Frustration
is different from confusion. A transfer is different from a long hold. A
rep hiding behind policy is different from a rep mis-setting expectations.
Until we tapped into conversational data, we were never able to measure the
additive effects of tonnage or intensity. Does it matter if a customer gets
frustrated three times in a call, as opposed to just once? Where does it
tip the scales from annoyance into actual churn risk? Without that field
chalked, without that nuance in measuring, we’d never get that from any
other method, survey or otherwise.
When all was said and done, the initial version of the our model, which
we’ve called the “Tethr Effort Index” is based on more than 400
variables together representing thousands of discrete phrases and
utterances that proved to be statistically significant in either increasing
or reducing effort. As we’ve been taking it out for a spin, it has proven
to be incredibly accurate in predicting the Customer Effort Score
that a customer would have given on a post-interaction survey, but
(of course) without having to ask the customer to fill out a survey.
Armed with a predictive Effort score on every customer call, a CX or
contact center leader can now track Effort levels in real time, immediately
drilling into those high-effort interactions that are likely to create
disloyalty and churn. Plus, with all of the rich verbatim from actual
customer interactions, leaders can quickly pinpoint, with tremendous
precision, the biggest opportunities for improvement in the customer
experience—whether it’s a change to a digital channel like the app or
website, a product fix, a call handling process change or an opportunity to
coach agents on new skills and behaviors. And, best of all, companies can
finally ween themselves from the post-call survey.
It's a brave new world out there!