By
Andrew Neff
|
Date Published: February 12, 2019 - Last Updated December 03, 2019
|
Comments
Chatbots are increasingly playing a role in customer service strategy. Their personalization and automation of some issues allow human agents more time to focus on the unique, difficult ones that they handle well. This blending of talents leverages the best of human and virtual agents, resulting in better overall service. As the contact center world focuses on metrics and KPI's, sector leaders have now developed measures to track chatbot success and are creating new ones every day. 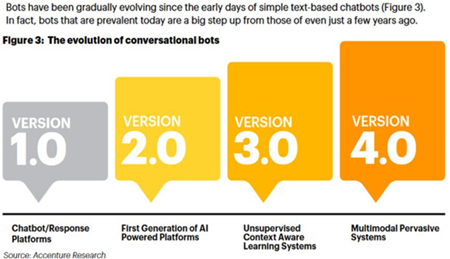
A Noteworthy Trend in Chatbots…and the Metrics
Until lately, there had been little delineation on the metrics spectrum from basic Chatbots up to complex AI Virtual Assistants. This trend is changing. Basic and more capable Chatbots can handle FAQ's or manage a transaction. As AI and Deep Learning expand their impact, the more sophisticated, conversational virtual agents can create full dialogues with customers, resolve multiple issues, understand the context and forward all necessary information to a human agent when a handoff is needed.
This evolution in bots, as noted here by Accenture Research, is leading to all new chatbot metrics that dive deeper into bots and their performance. 'Confusion trigger' and 'learning rate' are two examples that we'll explain, but let's start with metrics already being used today to track chatbot performance within overall customer service efficacy.
Today's Top 10 Chatbot Metrics
Deloitte Digital sites that "organizations are rapidly leveraging artificial intelligence to change the way they interact with customers dramatically". With the adoption of AI and chatbots, customer service management is still focused on delivering optimal service as priority one. This work means maximizing CSAT, NPS, agent retention and efficiency while keeping costs under control as the number of channels they use keep expanding. As always, tracking KPI's are a must to determine which goals are being met. Below are the top 10 chatbot metrics already being used today. Some correlate with traditional metrics while others are unique to chatbots. Since these metrics are newer, they often have different names, which are noted below.
1. Total Users: Defines your total number of Chatbot users during a time period. Just define a time period (common periods are 1, 3, 6 and 12 months) and track over time. Other accepted names include Users or User Rate.
2. New Users: Defines your acquired bot user numbers during a time period. Simply define a time period (common periods are 1 week and 1, 3, 6 and 12 months). Other names include Activation Rate or Acquired Users.
3. Active Users: Defines your return of frequent bot users within a certain time period. Simply define a time period (common periods are 1 week and 1, 3, 6 and 12 months). Other names include Retention Rate or Recurring Users.
4. Sessions: Defines how frequently users are interacting with the Chatbot. Similar time periods apply, although they tend to be shorter. Can be applied to one user or all. In the latter case, it is used to track how many times a bot on one page or one site is used within a time period, typically per day. Other names include Conversations, Total Conversations, Interactions or Interactions Per User.
5. Messages: Defines how many back-and-forth comments are made in one Chatbot session. This one is standard but needs perspective as different variables impact this measure. It could vary based on the bot's capability, the complexity of the inquiry, or the personality of the user (chatty or not). Other names include Turns, In-Messages, Bot Messages or Conversation Steps.
6. Average Handle Time (AHT): How long the Chatbot session lasts; but debatable as so many variables can impact the metric. Not only can the complexity of the inquiry effect it, but if the user is satisfied, they may start a second topic, which extends duration because the user is satisfied. Not many variations for this CCTR classic, but another name is Average Session Time. Note that agent AHT may increase slightly as bots often handle a higher percentage of the simple inquiries.
7. Topic: How many questions or transactions recovered in one Chatbot session. Similar to an IVR call, the user may start with one inquiry but extend to multiple inquiries during the session. More topics can be a good sign as the user has confidence in the Chatbot. Other names include Inquiries, Issues or Subjects.
8. Goal Completion Rate (GCR): Defines when the user's request has been satisfied by the Chatbot. Could be a question answered successfully of a transaction completed. Other names include Closure Rate, Completed Session, Completed Transaction, Successful Inquiry, Self-Service Rate.
9. Failure Rate: When the Chatbot cannot complete or does not understand the inquiry, and it must be escalated to a human agent. Can be triggered by the Chatbot truly not understanding, or less frequently, purposely triggered when the user offers up a certain inquiry that the company feels needs to be handled by an agent. Other names include Escalation, Failure, Elevation to an Agent, Fall Back Rate (FBR).
10. Customer Satisfaction (CSAT): Was the user satisfied with the bot UX and to what degree regardless of whether the inquiry or transaction was 100% completed? A CCTR industry standard, so there are few variations on the name.
4 New and Emerging Chatbot Metrics
Even as new metrics emerge, human agents remain crucial as ever. Chatbots and virtual agents are best used in combination with current service agents. As Forrester Research notes, "Don't replace your human service and sales teams - augment them." With agent/bot blending in customer service becoming more common, and chatbots using AI to evolve further and handle elements like topic switching and human sentiment, several new metrics are getting discussed and debated by customer service executives. Here are four examples:
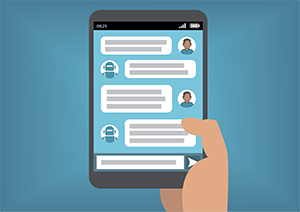
1. Confusion Trigger - Not popular yet, but growing. Measures either what caused the Chatbot session to end unsuccessfully or how many questions a Chatbot had to ask to get clarification on the user intent. Other names include Confusion, Recalibration Rate.
2. Learning Rate - Is the bot using AI and machine learning to improve performance over time so that issues that may have resulted in escalation three months ago no longer do? AI and learning algorithms are supposed to power this, but tracking the results show if that's indeed happening. Also known as AI Rate, Machine Learning Rate, Learning Improvement.
3. Revenue Growth or Transactions - How much revenue was generated per chatbot session tracked over a time period? This measure will be critical in some vertical sectors (e.g., Retail, Travel, Finance) and not as important to others focusing more on issue resolution.
4. Cost Savings - This starts to get into the world of internal metrics tracked. This basic one determines the net savings after subtracting costs for the Chatbot from the savings generated by reductions in total agent session time. Periods can vary from week to month to year and is usually tracked together with CSAT to measure the full impact. Also known as Customer Support Savings.
Sources:
1. "Chatbots Are Here To Stay," Accenture Research, May 2018
2. "Adopting The Power Of Conversational UI," Deloitte Digital, 2017
3. "Predictions 2019: Customer Service and Sales", Forrester Research, November 2018