By
Matt Dixon
|
Date Published: February 27, 2019 - Last Updated December 03, 2019
|
Comments
Quality Assurance, or QA for short, is in desperate need of repair in most companies. According to a recent study by research firm CEB, now a part of Gartner, only 12% of service leaders believe that their QA process is effective. But to understand the right way to fix QA, we first need to understand what's wrong with it.
QA, of course, is the process whereby companies "audit" calls for how well a representative adheres to company-defined scripting and language during a customer interaction. Did the rep use the customer's name? Did the rep thank the customer for her loyalty? Did the rep read the required disclosure statements after executing the transaction? Did the rep display empathy, friendliness, and professionalism? These audits help companies to ensure compliance and target training and coaching where needed. And, in many organizations, the output will feed performance evaluations and, ultimately, bonus compensation for reps.
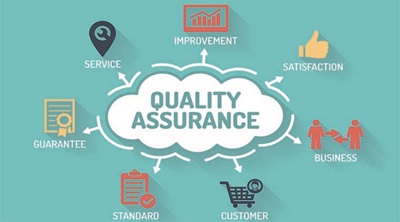
Few would disagree that the primary challenge facing QA organizations is the small sample size with which they have to work. Because QA is such a manual process (QA managers listening to and scoring calls), the reality is that for most companies QA will only ever listen to less than one percent of total call volume. This small sample size begets many other downstream issues.
First and foremost, reps often feel that the calls they've been scored on aren't an accurate reflection of their entire body of work. And, reps will often lament that QA managers, because they are people, can sometimes let their own biases slip into the grading process. This is why most companies have a separate "appeals process" for reps to have their scores and results reviewed to ensure that they are actually representative of their performance.
But the bigger issue isn't that reps think QA is unfair (which is certainly an issue as it negatively impacts morale and the work environment), but that leaders, as reflected in the CEB/Gartner survey, don't believe QA actually improves the quality of service interactions. As one QA manager admitted to me at a recent conference, "We change our QA scorecard every month…we keep trying to 'crack the code' on the things that actually drive quality and customer satisfaction. But for all of the changes we've made, we really haven't moved the needle much, if at all."
For customer service leaders, recent advances in technology hold a lot of promise to change the QA playing field fundamentally. With the help of transcription engines and sophisticated machine learning platforms, companies can now have machines "listen" to a far greater number of calls than a team of QA managers ever could. No more heavy labor investment. No more limited sample sizes. No more appeals process for reps who believe their scores don't accurately reflect their work. No more human bias. Sounds great, right?
Not so fast. Many companies have been quick to jump on this bandwagon, automating QA using AI-powered speech analytics. But in our experience, this can often make things worse, not better.
The real issue with QA isn't that it's inefficient and expensive (which it is), but that it tends to be highly assumption-driven. Companies ask QA to listen for things they think are essential (e.g., saying the company's name at the beginning of a call for brand association or saying the customer's name multiple times to make the customer feel the interaction is personalized). But these assumptions, regardless of how well-intentioned, have rarely been tested with data. This is why when most companies regularly update their QA scorecards-without a compass to show them where to go, they resort to guessing.
Join Matt this May 13-16 at ICMI Contact Center Expo, where he'll be speaking in three sessions.
If companies don't first do their homework to understand what is actually driving quality, they will become more efficient…but no more effective. Without knowing whether what's on your QA scorecard even matters, companies run the real risk of automating bad processes-effectively, scaling mediocrity or even driving performance in the wrong direction.
The best companies are using the latest advanced technology to finally answer the age-old question of what leads to a high-quality customer experience. Armed with machine learning and data science techniques, these companies are seizing upon the opportunity to finally overhaul call center QA so that it delivers what it was initially intended to deliver: higher quality customer interactions.
Here's how one company we work with tackled this challenge.
This company, a large home services provider, was looking to automate QA using AI, but first wanted to use the technology to understand what's driving the outcomes they care about like CSAT, NPS or Customer Effort Score. To figure this out, they first transcribed all of their calls-effectively transforming their call recordings from unstructured audio into unstructured text. Next, they brought structure to the text data by "categorizing" it. Working with our team and their own QA group, they created machine learning training sets (also called categories) that captured the way each of the skills and behaviors from their QA scorecard manifest in terms of language.
For instance, one of the behaviors they always assessed their reps on was advocacy-i.e., when reps use language designed to show the customer that they're on the customer's side and are going to work to get to a positive resolution of the issue. Our team had broken advocacy down into more than 130 different "utterances" that a rep could conceivably use. The company did this for all 15 of the behaviors they assess reps on with their QA scorecard. The unstructured text was then tagged with these different machine learning categories so that the company knew exactly which calls their reps had effectively demonstrated the behaviors on and which they hadn't.
Next, the company matched up the individual calls with completed post-call surveys as well as the other outcomes they care about like sales conversion. With our help, they built a regression model for understanding the impact of their 15 QA scorecard behaviors on these outcomes, finally revealing whether the things they'd always told their reps were important were actually driving the outcomes the business cared about.
It was an eye-opening analysis.
First, the company found more than half of the things on their scorecard-some of which they had preached through training and coaching for years (e.g., thanking the customer for her loyalty)-had no bearing on their key business metrics. As the head of QA told us, "At the end of the day, it turns out that some of the things we require our reps to say during customer calls may have made us feel good, but our customers couldn't have cared less."
Second, they found that some of the things they'd always preached-like acknowledging the customer's issue and expressing empathy-only worked when coupled with other behaviors like advocacy. When reps acknowledged a customer's pain but didn't immediately take ownership of the problem and drive to a resolution, the effect was actually negative-as bad as transferring a customer to a different department.
They also learned that some of the behaviors they taught in their training and coaching sessions, such as advocacy, must be applied differently in different contexts. In a sales context, advocacy is best demonstrated when a rep confidently guides the customer to the right offer (e.g., "I've got the perfect service package for you") but that level of confidence backfires in service situations. When customers are experiencing an issue, it's far better not to be as declarative (e.g., "I've got a few ideas for how to fix this issue…let's try this one first").
Finally, they learned that while the correct, contextualized use of certain techniques could have a positive impact on key outcomes, it was equally (if not more) important to look for the opposite of these behaviors. For instance, the opposite of advocacy is when reps hide behind policy (what we call "powerless to help" language), and using this sort of language is actually far more detrimental to the customer experience than not demonstrating advocacy at all. Unfortunately, their QA team had never listened for this sort of language in the past, overlooking a vast opportunity to engineer a better experience for customers.
Armed with this insight, the company completely overhauled its QA scorecard and was able to use machine learning to score all calls against the new, revised set of criteria. In the end, the QA team-freed from the tedium of manually listening to calls-was redeployed to coach reps on how to reach higher levels of competence on each of the behaviors the company now knew mattered to driving quality.
AI is a super exciting technology that holds the potential for transforming much of what we do in customer service. But we need to be thoughtful about how we deploy it so that we're actually getting better, not just automating bad processes. Beware of anyone telling you they can automate QA for your organization without first doing the critical work of understanding what drives quality in your organization.
Our strong advice to service leaders is to "fix first, then automate," not the other way around.